-
Published
2019-03-20
-
Author
Clain Team
- Topics Investigations
- Share
As part of routine exercise in analyzing the prominent crypto hacks, we occasionally share our findings with public and invite interested parties to collaborate.
Clain is engaged in developing tools for compliance solutions in decentralized networks. By using a combination of network science and machine learning to aggregate and interpret vast quantities of transactional data, we provide investigative services to participants of cryptocurrency ecosystem and give them a meaningful insight of the network’s internals.
We believe cryptocurrencies will play a significant role in the future and, therefore, we care deeply about improving transparency and endorse adoption of cryptocurrencies as a legitimate means of value exchange.
What happened
In September 2018 at 11:33 UTC time the Japanese based crypto exchange Zaif was hacked and roughly 5957.6 BTC were transferred to 1FmwHh6pgkf4meCMoqo8fHH3GNRF571f9w address in multiple rounds within a short period of time. From this on, the stolen bitcoins moved further in smaller chunks to dozens of transient addresses for obfuscation.
What we found out
We were able to identify at least 1461 BTC addresses affiliated with hackers (full list of addresses with balances as of 15 February 2019 is attached to the link). Yet, we believe the ultimate number of addresses involved in this crime might reach over two thousand as we left some addresses unverified until now.
As we progressed with our analysis, we detected that at least 875,75 BTC had been laundered through ChipMixer, and at least 1549,31 BTC landed into Binance deposit addresses predominantly in small fractions of 1,9 BTC, which is the maximum allowed to withdraw from unverified accounts.
Timing wise, the transactions headed to Binance occurred mostly during the first days of the crime, while the portion of funds laundered through Chipmixer took a longer time frame.
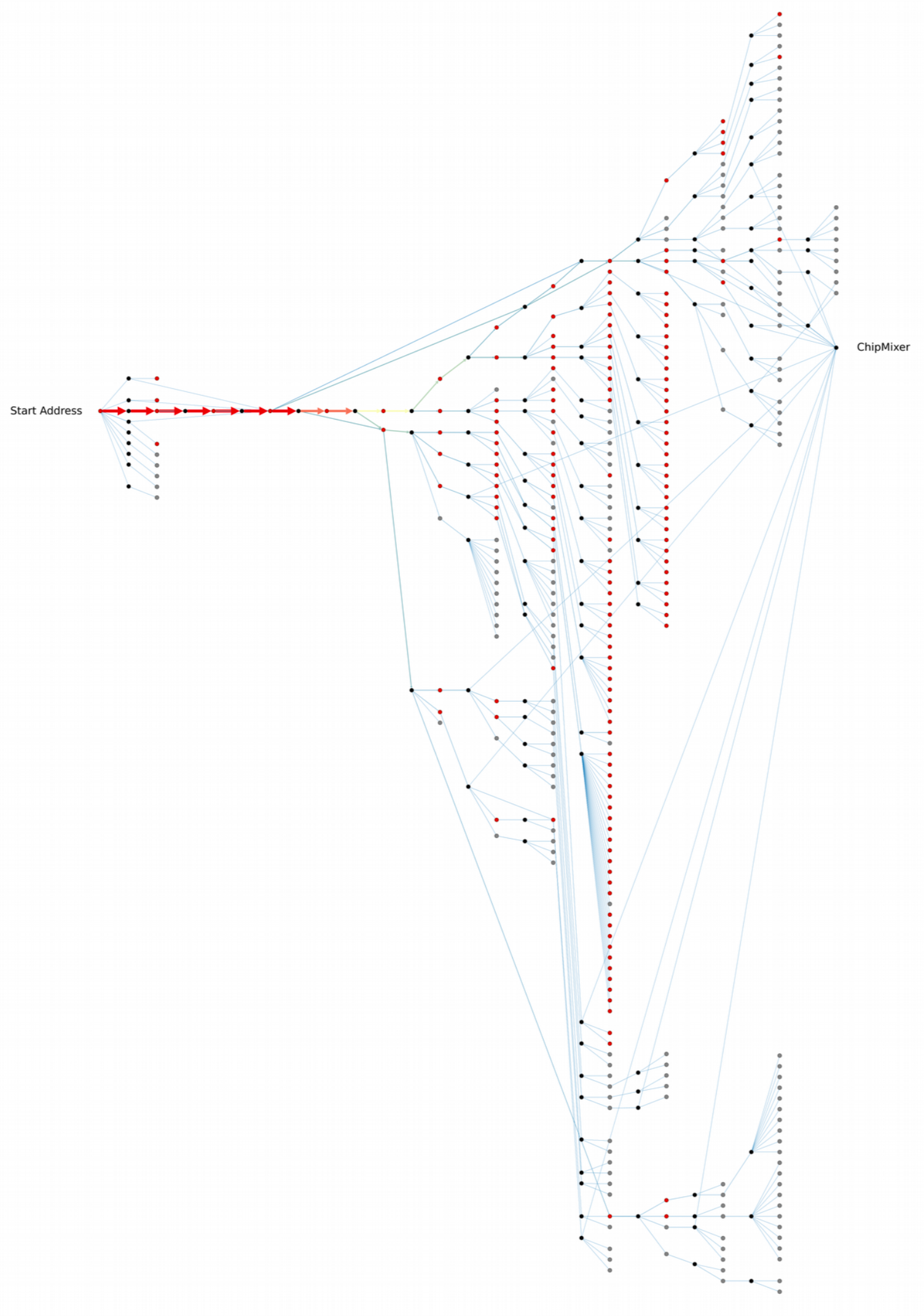
In case of Binance, we spotted at least 83 transactions with 402 unique addressed used to deposit the stolen funds. The number of such magnitude implies that the hackers are likely used automated scripts to create dozens of Binance fake accounts to maximize laundering throughput and remained unnoticed.
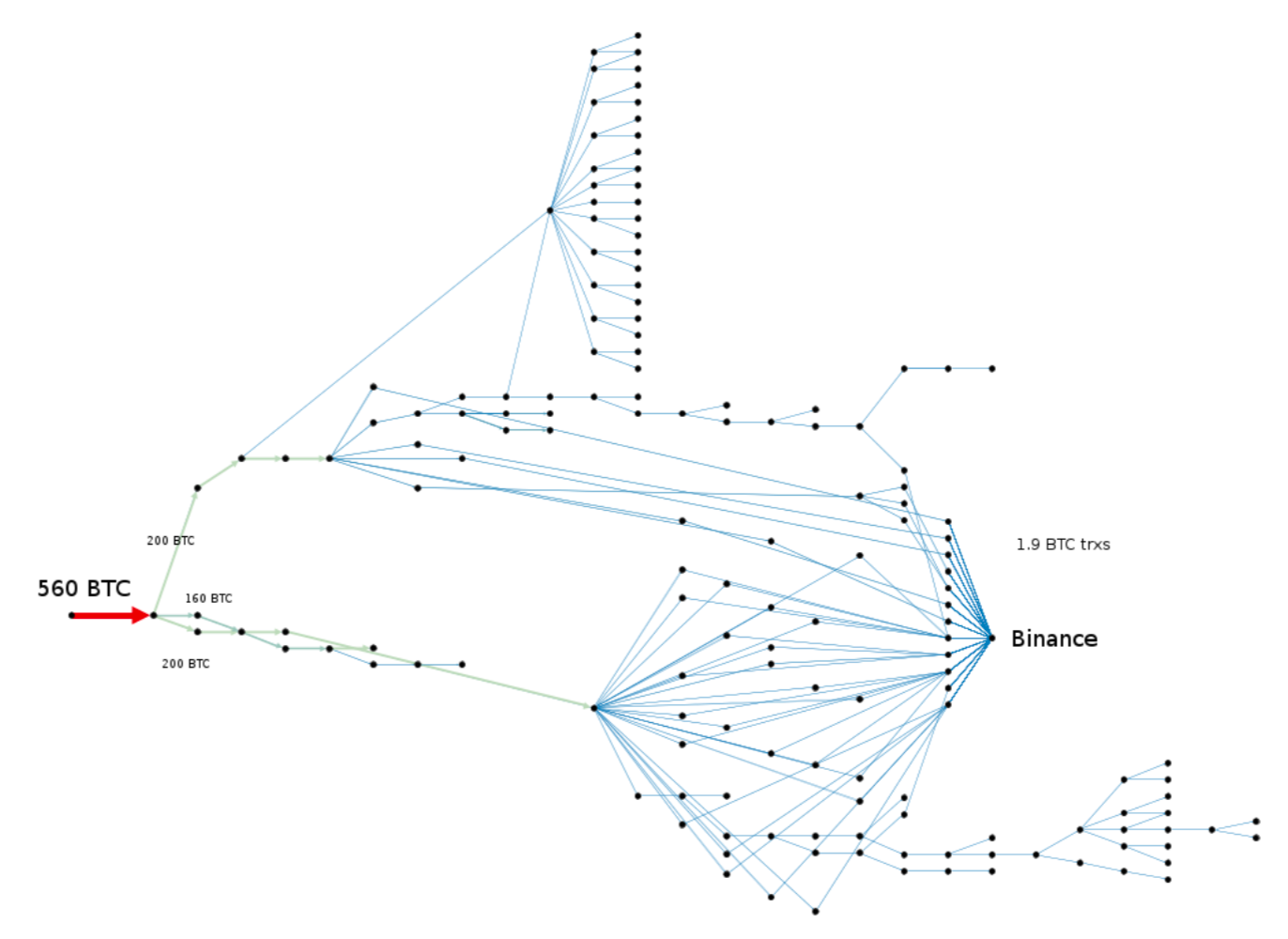
We concluded that at least 40% of the stolen money was laundered through ChipMixer and Binance, and about 60% is still scattered across hackers addresses that we know little about.
How we found out
When hackers commit crimes to steal crypto, they typically create a large number of chain transactions by splitting and aggregating the stolen funds into newly created addresses with multiple iterations in order to obfuscate the illicit source. The ultimate goal of the hackers, often but not always, is to exchange the laundered proceeds to fiat currencies.
To better visualize the major flows of the stolen bitcoins, at first, we normally screen for transactions with significant notional values (in this particular case we applied filter for 50 BTC and higher). A bigger picture revealed further splits into chunks that, as our internal investigation concluded, eventually headed to ChipMixer and Binance for laundering.
ChipMixer is a version of cryptocurrency mixing services that has a distinctive feature. To send out laundered funds, the mixer creates chips with nominal values of 0,001BTC; 0,002BTC; 0,004BTC; 0,008BTC and so forth up to 8,192BTC. By knowing that mixing transactions have strict value rules, we created the algorithms that quickly allowed us to spot the suspicious transactions with great confidence.
Hackers attempt to create hundreds of transient addresses to make stolen funds appear indistinctive to a human eye. However, the attempts typically reveal itself if put through the lens of a carefully crafted model, able to detect the shared attributes of illicit transactions and their inherited changes with each passing transfer. The model allowed us to spot the shift in the hacker's software used to sign in transactions or detect a change in the ownership.
Below is a 2-dimentional visual representation of those attributes showing that dots with closest proximity to each other are sharing the same features.
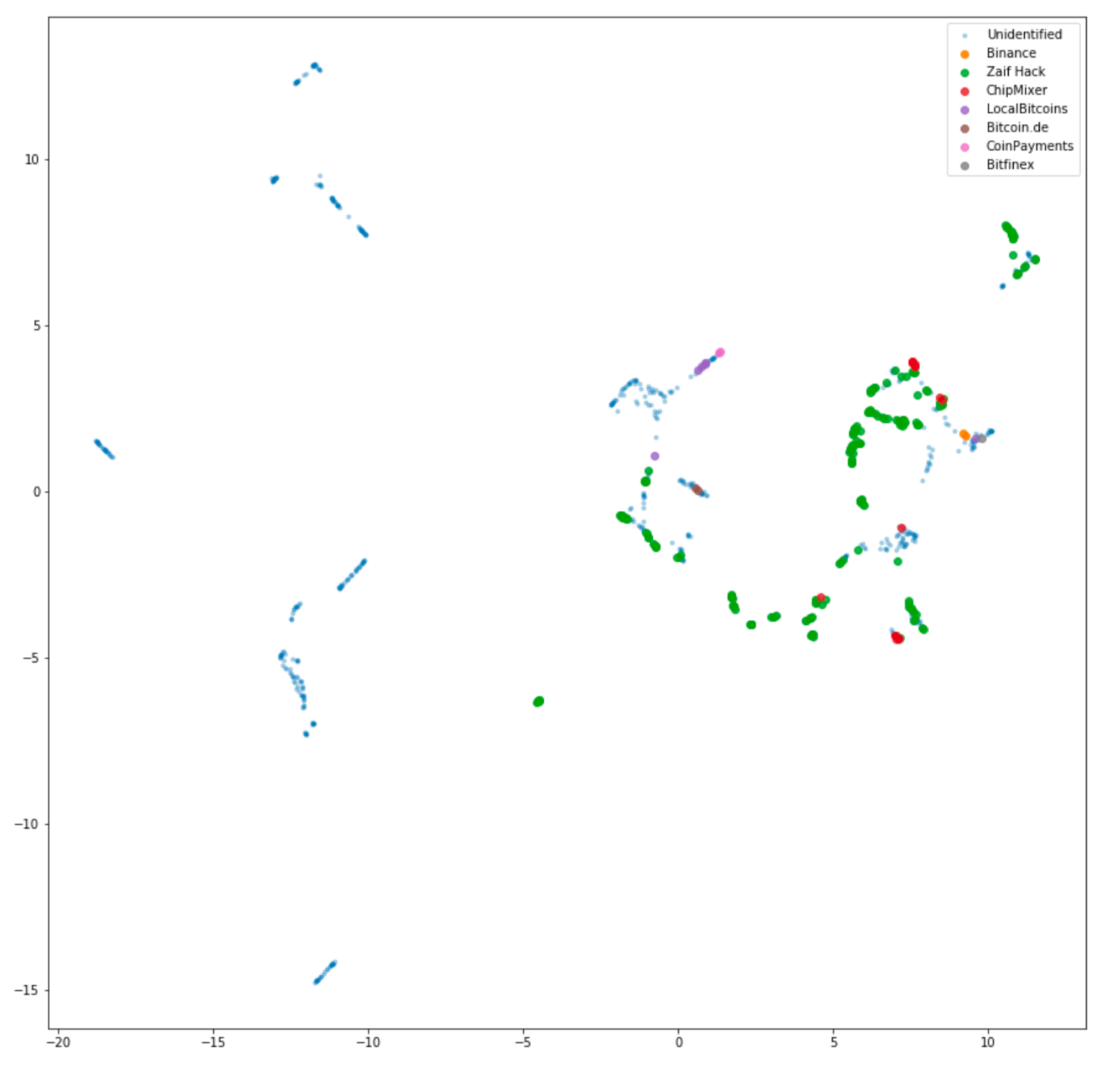
We have successfully applied our models to perform tasks of detecting and classifying addresses by various attributes and statistics including time intervals. Once we obtained the sufficient number of accurately tagged hacker’s addresses, we applied supervised machine learning to spot the additional number of involved addresses, the full list of which we share below.
Our platform works real-time and enables to monitor cases on a continuous basis as new relevant data pops up. If we happen to learn further significant evidence to this current investigation, we will update our analysis in the due course.